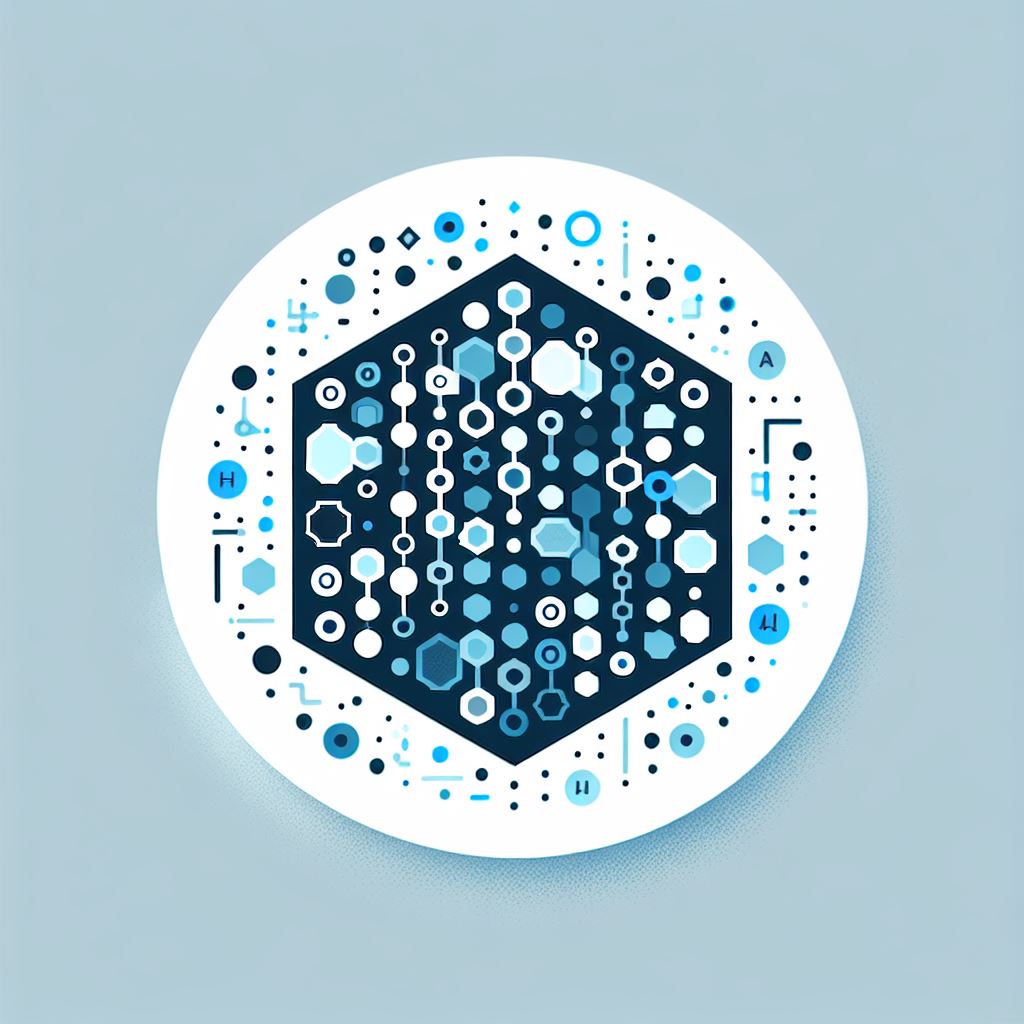
Data-centric AI
Data-centric AI is defined as “the discipline of systematically engineering the data needed to build a successful AI system.” and is concerned with real-world data that are unstructured, often incomplete/limited in number, and partially inconsistent. At ISGroup, we actively work on real-world case studies and datasets from diverse application areas, including clinical medicine, human resource management, and industrial production. Our goal is to develop tailored data preparation and AI methodologies that effectively handle data in the wild, ensuring robust and reliable AI performance in these challenging environments. We aim to create AI solutions that meet the unique requirements of each application context, making AI both practical and stable in real-world settings.
Funded Projects
WASABI: White-label shop for digital intelligent assistance and human-AI collaboration in manufacturing
HybridAI: an hybrid approach to Natural Language Understanding
Work Datafication and Behavioral Visibility in The Digital Workplace
Assessment of well-being state in Post-acute COVID Syndrome: an international multicentre prospective cohort study
Machine Learning to Operationalize WG definition and Generate a Personalized Phenotypic Characterization in PLWHIV
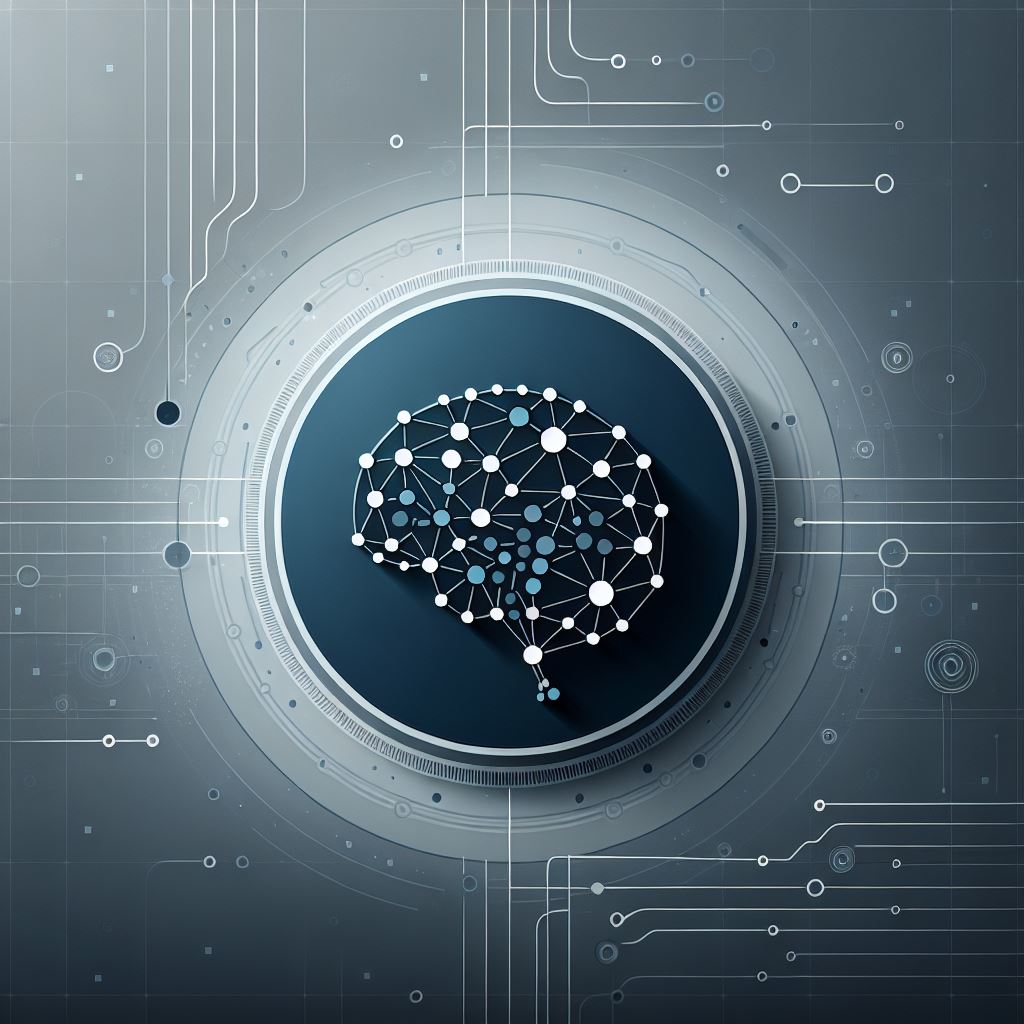
Interpretable AI
In high-stakes domains such as healthcare and finance, the need for interpretable AI has become paramount. At ISGroup, we specialize in developing symbolic machine learning and multicriteria decision-making techniques that prioritize interpretability. These approaches offer significant advantages by providing domain experts with clear, understandable results, thereby enhancing their confidence in AI-driven solutions. This interpretability empowers experts to make well-informed decisions when selecting the most appropriate model, taking into account factors such as data availability, preferences, costs, and domain-specific understanding.
Funded Projects
Assessment of well-being state in Post-acute COVID Syndrome: an international multicentre prospective cohort study
Machine Learning to Operationalize WG definition and Generate a Personalized Phenotypic Characterization in PLWHIV
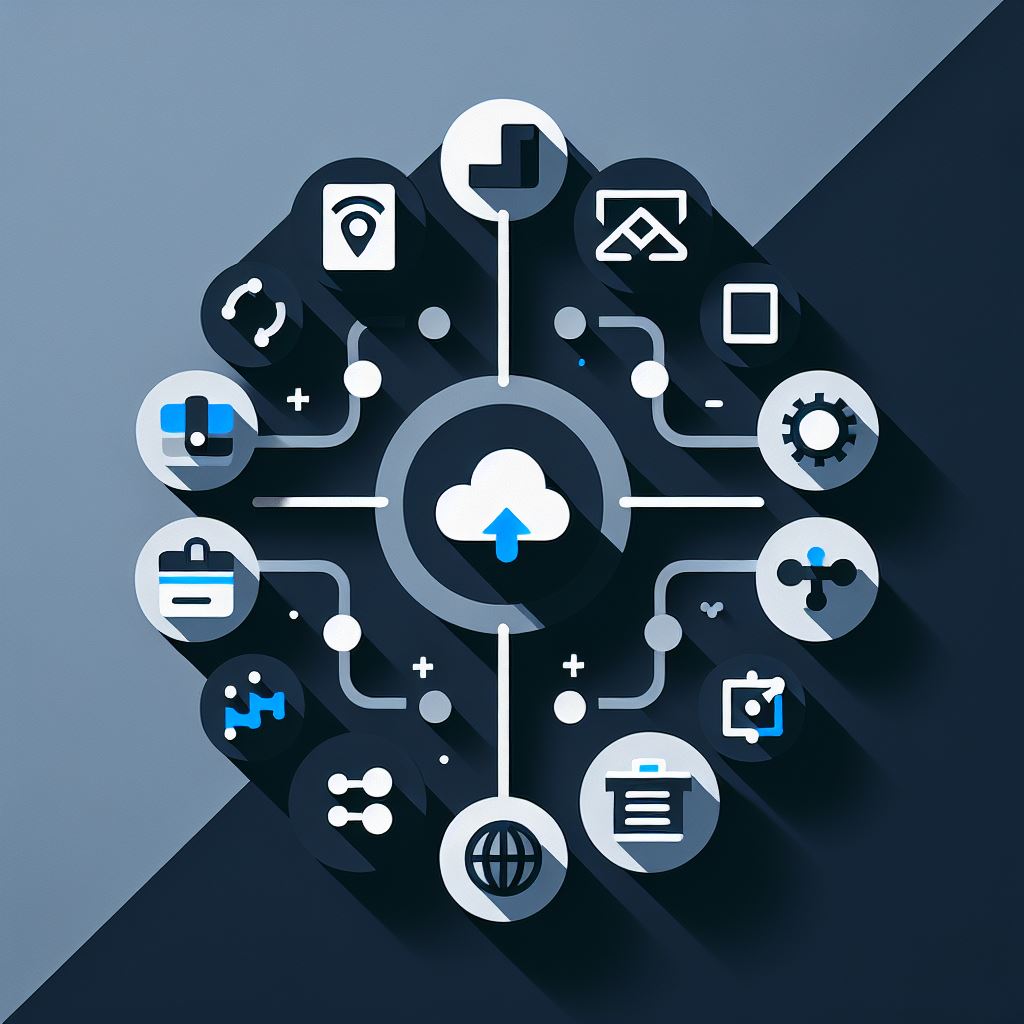
Information sharing, interoperability, and retrieval
The ever-growing and widespread availability of data from Internet information sources has placed great interest in the potential of information sharing and interoperability.
Our work in this field concerns the sharing and interoperability of heterogeneous and distributed data sources. It was first focused on the Peer-to-Peer (P2P) paradigm and then on its evolutions toward dataspaces and data lakes. We consider various application contexts, ranging from digital libraries to business intelligence and smart industry.
Funded Projects
NeP4B: Networked Peers for Business
WISDOM: Web Intelligent Search based on DOMain ontologies
DELOS – A Network of Excellence on Digital Libraries
Technologies and Services for Enhanced Content Delivery (ECD)
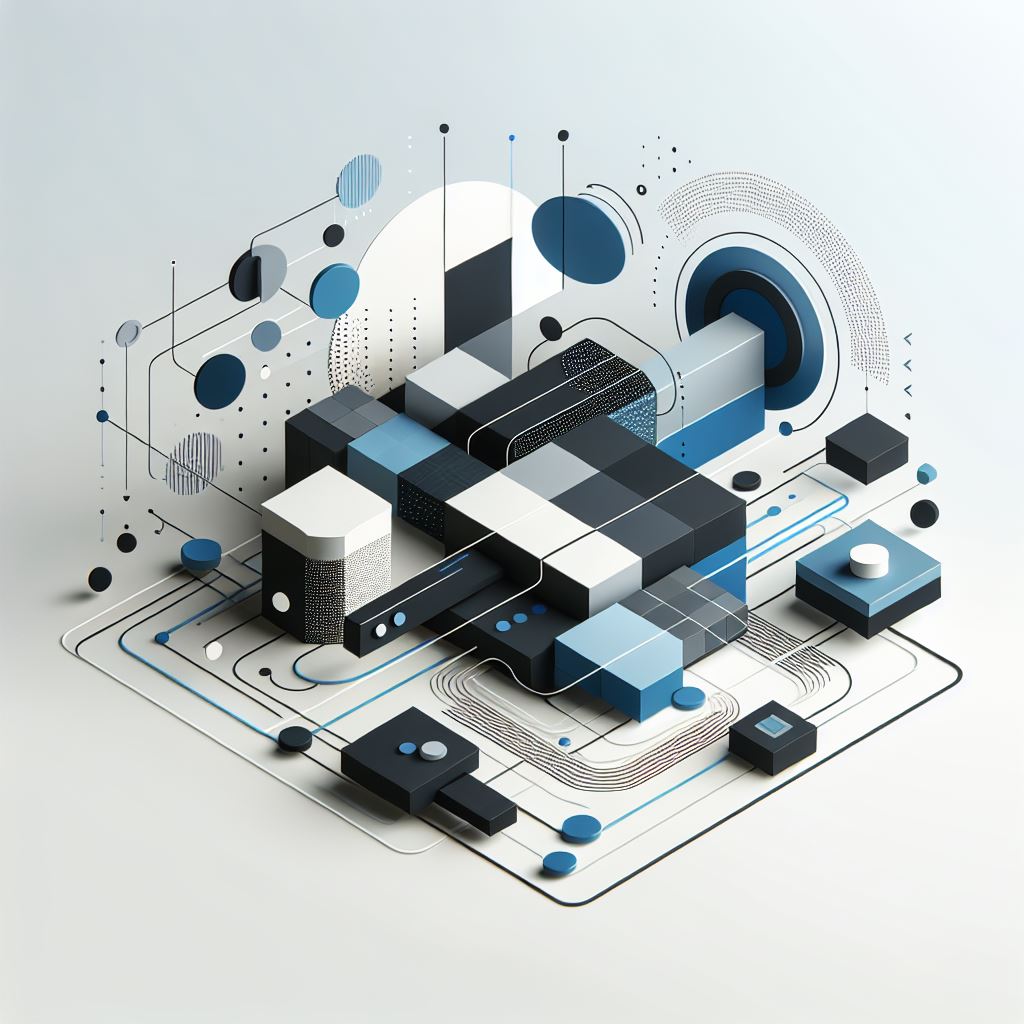
Non-conventional data management
We study solutions for the efficient management of different kinds of data, from data streams, including sensor data and RFID data, to textual and semi-structured data. We are especially interested in developing models and techniques for query processing in the context of workload-intensive applications.
Funded Projects
NeP4B: Networked Peers for Business
Pegasus
Outdoor Video Protection: innovative vision and information technologies for safe outdoors work environments